In the era of big data, organizations are inundated with vast amounts of information from various sources. Managing and deriving value from this data can be challenging, but data lakes have emerged as a powerful solution. Data lakes offer a flexible and scalable approach to storing and analyzing large volumes of data. However, like any technology, they come with their own set of benefits and challenges. In this article, we’ll dive into what data lakes are, their advantages, and the potential hurdles organizations might face when implementing them.
What is a Data Lake?
A data lake is a centralized repository that allows organizations to store all their structured and unstructured data at scale. Unlike traditional data warehouses, which store data in a structured format, data lakes can handle raw, unprocessed data from diverse sources, including social media, IoT devices, and transactional systems.
Data lakes use distributed storage systems to accommodate the vast amounts of data they manage. This approach provides flexibility and scalability, allowing organizations to ingest, store, and analyze data without the need for predefined schemas or data models.
Key Benefits of Data Lakes
- Scalability and FlexibilityOne of the most significant advantages of a data lake is its scalability. Data lakes are designed to handle massive volumes of data, making them suitable for organizations with large and growing data sets. The distributed architecture allows for easy scaling, both in terms of storage capacity and computational power. Additionally, data lakes offer flexibility in data ingestion. They can accommodate various types of data—structured, semi-structured, and unstructured—without requiring predefined schemas. This flexibility allows organizations to store raw data and process it as needed, providing a versatile solution for different data types.
- Cost-Effective StorageData lakes often leverage low-cost storage solutions, such as cloud-based object storage systems. These systems are generally cheaper than traditional data warehouses and allow organizations to store large volumes of data economically. By avoiding the need for expensive storage hardware, organizations can reduce their overall data management costs. Moreover, cloud-based data lakes offer pay-as-you-go pricing models, which means organizations only pay for the storage and processing resources they use. This cost-effectiveness makes data lakes an attractive option for organizations looking to manage their data efficiently without breaking the bank.
- Enhanced Data IntegrationData lakes facilitate the integration of data from disparate sources. By storing data in its raw form, organizations can combine information from various systems and applications without the need for extensive data transformation or preprocessing. This capability is particularly useful for organizations with diverse data sources, such as customer interactions, sales transactions, and sensor data. By integrating all this information into a single repository, organizations can gain a holistic view of their operations and make more informed decisions.
- Advanced Analytics and Machine LearningData lakes enable advanced analytics and machine learning by providing a comprehensive data repository. Data scientists and analysts can access vast amounts of raw data to build predictive models, perform complex queries, and derive actionable insights. With the right tools and technologies, organizations can leverage data lakes for real-time analytics, big data processing, and machine learning applications. This capability allows for more sophisticated data analysis and enables organizations to uncover trends and patterns that might not be visible with traditional data storage methods.
- Future-Proofing Data ManagementAs data continues to grow in volume and complexity, data lakes offer a future-proof solution for managing and analyzing information. Their scalability and flexibility make them well-suited to accommodate evolving data needs and technological advancements. By adopting a data lake approach, organizations can ensure they are prepared for future data challenges and opportunities. This proactive strategy allows them to stay ahead of the curve and adapt to changing business requirements and technological developments.
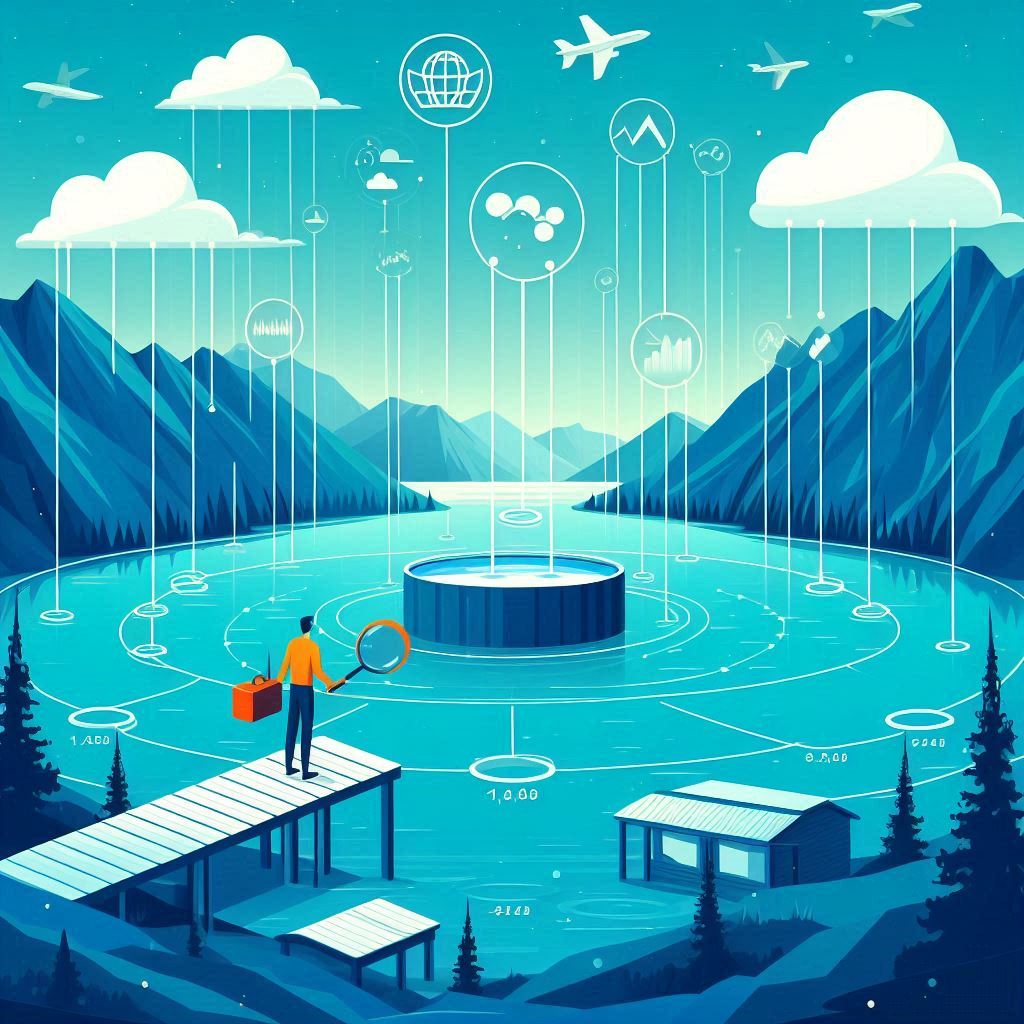
Challenges of Data Lakes
- Data Governance and QualityWhile data lakes offer flexibility in data storage, they also present challenges related to data governance and quality. Storing raw, unprocessed data can lead to issues with data consistency, accuracy, and completeness. Without proper data governance practices, data lakes can become chaotic repositories of unstructured data, making it difficult to ensure data quality and integrity. Organizations need to implement robust data management strategies, including metadata management, data cataloging, and data validation, to address these challenges effectively.
- Complex Data ManagementManaging a data lake requires sophisticated tools and processes to handle the volume, variety, and velocity of data. Organizations must invest in technologies and expertise to effectively store, process, and analyze data within the lake. Additionally, as data lakes grow, the complexity of managing and accessing data can increase. Organizations may face challenges related to data discovery, data integration, and query performance. Implementing effective data management practices and tools is essential to overcoming these complexities.
- Security and Privacy ConcernsData lakes often store sensitive and confidential information, making security and privacy a critical concern. Ensuring that data is protected from unauthorized access and breaches is paramount for maintaining trust and compliance. Organizations must implement stringent security measures, including encryption, access controls, and monitoring, to safeguard data within the lake. Additionally, they need to comply with relevant data privacy regulations, such as GDPR and CCPA, to protect personal information and avoid legal repercussions.
- Data Silos and FragmentationWhile data lakes aim to integrate data from various sources, there is a risk of creating data silos within the lake itself. Different teams or departments may store data in separate areas of the lake, leading to fragmentation and difficulties in accessing and analyzing data. To address this challenge, organizations should establish clear data management policies and practices that promote data integration and collaboration. Implementing centralized data cataloging and metadata management solutions can help reduce data silos and improve data accessibility.
- Performance and Latency IssuesThe performance of a data lake can be affected by the sheer volume of data and the complexity of queries. As data lakes grow, query performance and latency may become issues, impacting the speed and efficiency of data analysis. To mitigate these performance challenges, organizations can employ various optimization techniques, such as data partitioning, indexing, and caching. Additionally, leveraging cloud-based data lake solutions with built-in performance optimization features can help address latency and performance concerns.
Conclusion
Data lakes represent a powerful and flexible approach to managing and analyzing large volumes of data. They offer significant benefits, including scalability, cost-effective storage, enhanced data integration, and advanced analytics capabilities. However, organizations must also be aware of the challenges associated with data lakes, such as data governance, management complexity, security, and performance issues.
By understanding these benefits and challenges, organizations can make informed decisions about adopting data lakes and implement strategies to maximize their value while addressing potential hurdles. As data continues to play a crucial role in business success, leveraging data lakes effectively can provide a competitive edge and drive innovation in the data-driven era.